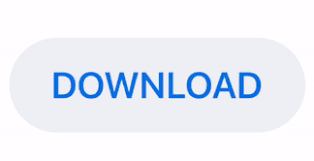

- #AVOID DISPLAYING DATA IN PLT.HIST PYTHON JUPYTER NOTEBOOK HOW TO#
- #AVOID DISPLAYING DATA IN PLT.HIST PYTHON JUPYTER NOTEBOOK INSTALL#
The example mentioned below illustrates the use of the above-mentioned terms: The axes are effectively the area that we plot data on. It can be created using the figure method of pyplot module.Īxes is where the plotting occurs. We can have multiple independent figures, and each figure can have multiple Axes. It is the overall window where everything is drawn. So let us begin by defining specific terminology used across the domain.įigure is the top-level container in the hierarchy. However, we will start learning the components and it should feel much smaller and approachable.ĭifferent sources use 'plot' to mean different things. Matplotlib is a large project and can seem daunting at first. Python Matplotlib allows creating a wide variety of plots and graphs. Before we start plotting graphs, let us first understand the key terms in the next section of the Python matplotlib tutorial.
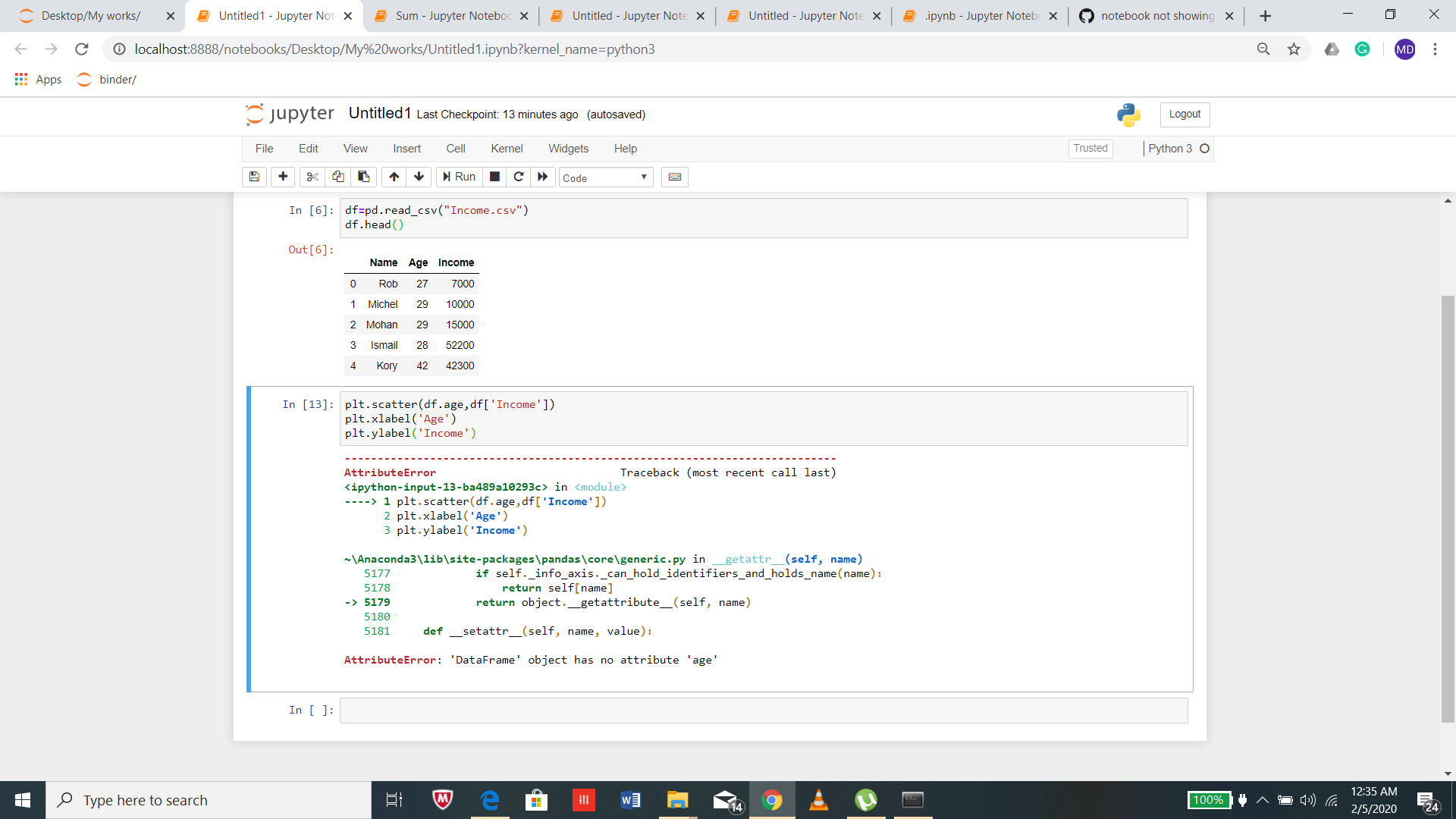
#AVOID DISPLAYING DATA IN PLT.HIST PYTHON JUPYTER NOTEBOOK HOW TO#
Thus, you have understood how to import matplotlib.
#AVOID DISPLAYING DATA IN PLT.HIST PYTHON JUPYTER NOTEBOOK INSTALL#
You can go through the following blog to understand how to install and import Python packages.
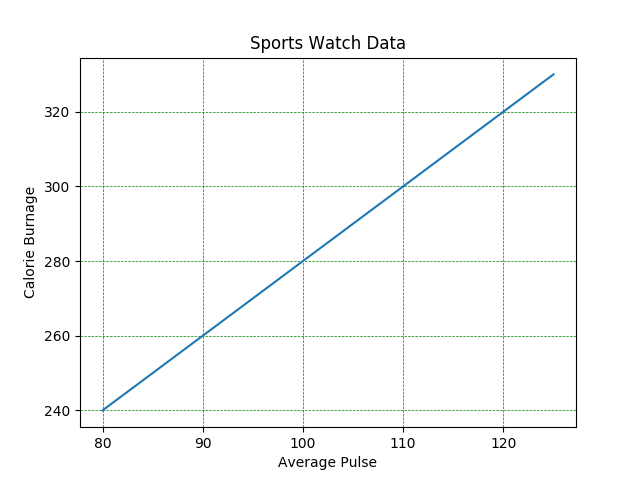
It can be installed using the command pip install matplotlib. Matplotlib do not fall under the Python Standard Library and hence, like any other third-party library, it needs to be installed before it can be used. Import matplotlib.pyplot as plt % matplotlib inline This is demonstrated in the example below: Also, if we are working in a Jupyter Notebook, the line %matplotlib inline becomes important, as it makes sure that the plots are embedded inside the notebook. The pyplot being the sub-package within Matplotlib provides the common charting functionality. It is a common practice to import matplotlib.pyplot using the alias as plt. To get started with Python Matplotlib, we first import the package.
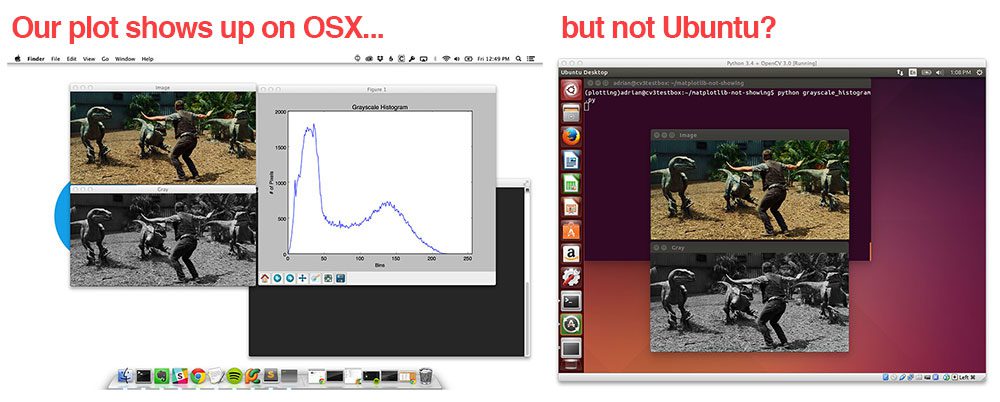
Machine Translation with Attention (Thushan) Machine Translation (Sequence-to-Sequence) Seqeunce Model with Attention for Addition Learning Sequence Model (many-to-one) with Attention Intutions for Types of Sequence-to-Sequence Models Generate Text Embeddings Using AutoEncoder Sentiment Analysis with Traditional Machine Learning Machine Learning with Sklearn – Regression Chinese Natural Language Processing (spaCy)
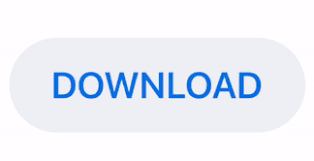